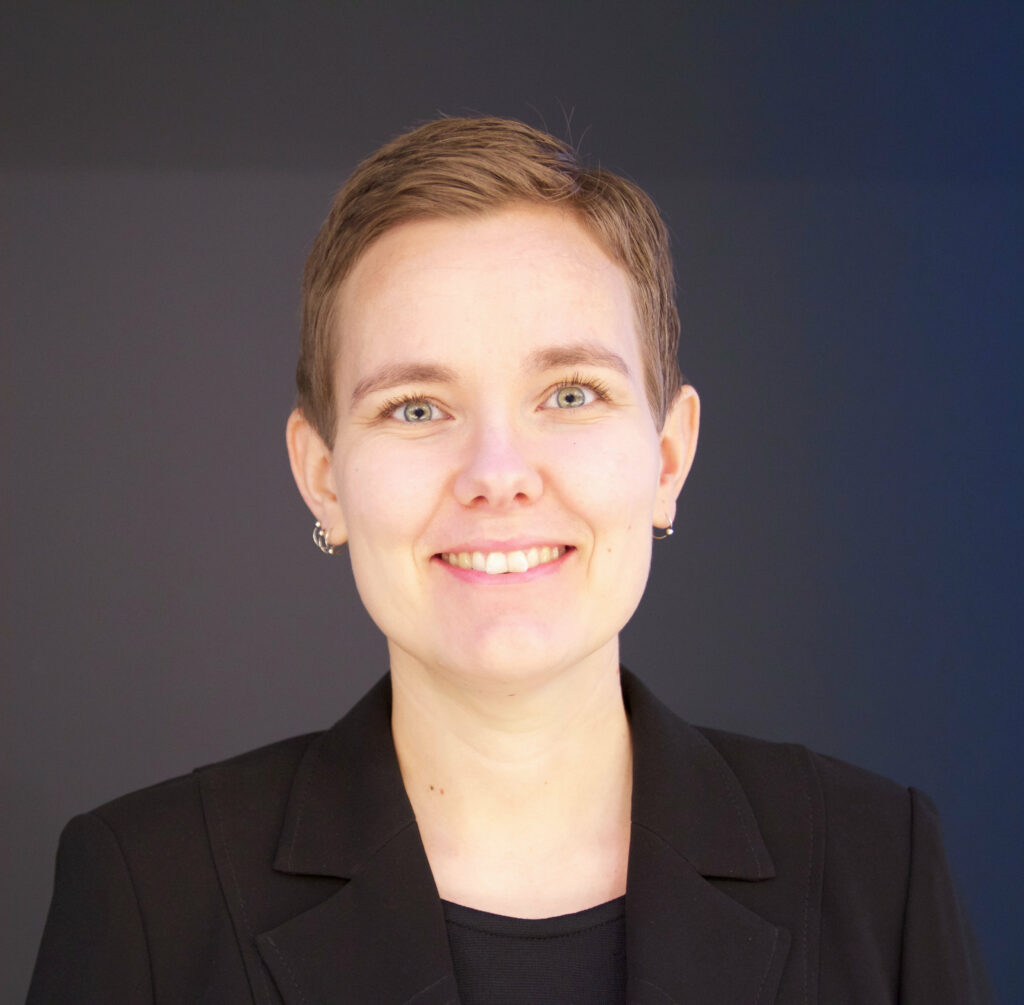
Topic: Computational tools for multi-level profiling of individual cancer risks
Speaker: Professor, Research Director, Laura Elo, Medical Bioinformatics Centre, Turku Bioscience Centre, Turun yliopisto & Åko Akademi
Get to know the speaker: https://elolab.utu.fi/
This time the FICAN Science Webinar is organised by FICAN West. The event is open for all interested in cancer research.
Abstract
Computational techniques are playing an increasingly important role in cancer research for interpreting complex molecular and clinical data, enabling novel insights into individual cancer risks. An interesting opportunity lies in the re-analysis of existing publicly available datasets with advanced statistical and machine learning approaches for new discoveries. Reproducibility is a critical area to ensure reliable findings that can eventually translate into clinical applications to advance cancer diagnostics and therapies.
Proteomics has emerged as a rich source of biomarkers, offering a more direct measure of the phenotype than genetic information. The development of new computational tools, like deep learning based de novo sequencing, is moving the interpretation of proteomics data to a new level, including from already generated datasets. By applying these tools to publicly available cancer datasets, such as those for breast cancer, enable discovery of new biomarker candidates with potential clinical applications.
At the same time, single-cell technologies are enhancing the depth of our analyses, providing detailed views on cellular heterogeneity and tumor microenvironment. The construction of comprehensive cell atlases through efforts in data integration enables studying cellular patterns and interactions associated with disease progression or treatment outcomes. For instance, construction of an immune cell atlas allows identifying risks of immune-related adverse events in patients undergoing immune checkpoint inhibitor therapy.
In addition to interpreting multi-level molecular profiles, machine learning provides various opportunities to predict disease and treatment risks from other types of digital health data, such as electronic health records. For example, prediction of chemotherapy-induced neutropenia risk can be improved with machine learning to facilitate more targeted use of granulocyte colony stimulating factors (G-CSFs).
Relevant references for this talk:
- Van Den Bossche T, Beslic D, van Puyenbroeck S, Suomi T, Holstein T, Martens L, Elo LL, Muth T. Metaproteomics beyond databases: addressing the challenges and potentials of de novo sequencing. DOI: 10.26434/chemrxiv-2024-4v6q0
- Smolander J, Junttila S, Elo LL. Cell-connectivity-guided trajectory inference from single-cell data. Bioinformatics 39(9):btad515, 2023.
- Välikangas T, Suomi T, Chandler CE, Scott AJ, Tran BQ, Ernst RK, Goodlett DR, Elo LL. Benchmarking tools for detecting longitudinal differential expression in proteomics data allows establishing a robust reproducibility optimization regression approach. Nat Commun 13(1):7877, 2022.
- Venäläinen MS, Heervä E, Hirvonen O, Saraei S, Suomi T, Mikkola T, Bärlund M, Jyrkkiö S, Laitinen T, Elo LL. Improved risk prediction of chemotherapy-induced neutropenia – model development and validation with real-world data. Cancer Med 11(3):654-663, 2022.